From Data to Decisions: Extracting Value from Energy and Commodities Data
- Ana Jesus
- Mar 25
- 6 min read
Updated: Mar 27
Navigating the Data Deluge in the Digital Age
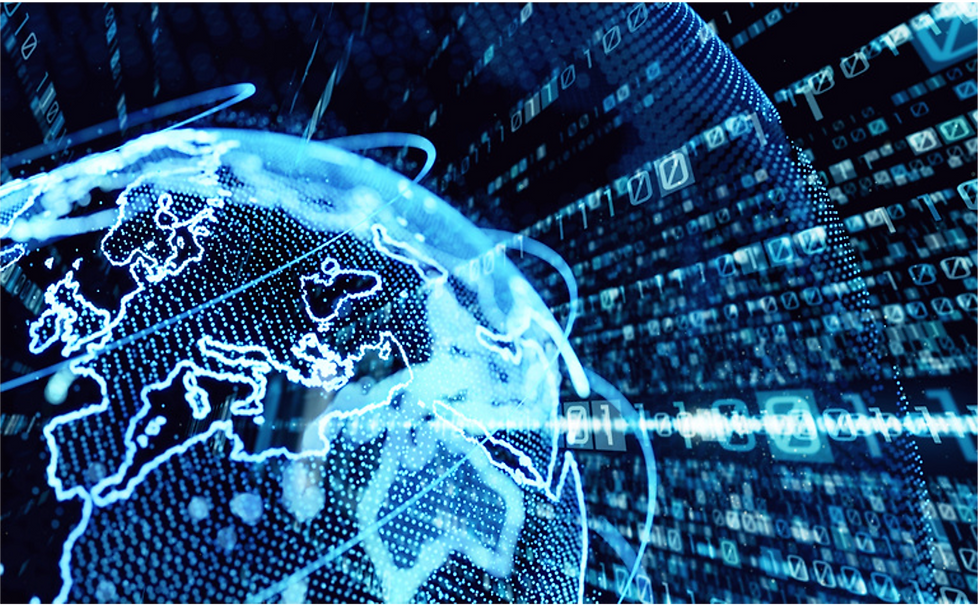
The energy and commodities sectors are experiencing a period of unprecedented complexity and opportunity. A relentless data deluge fed by real-time market feeds, sensor data from drilling operations, fluctuating weather patterns, unpredictable geopolitical events, and evolving consumer demand is reshaping the industry. This abundance of information, while offering unparalleled potential, presents a significant challenge: how to transform this vast ocean of data into actionable insights, strategic advantages, and ultimately, increased profitability. The digital revolution, powered by cloud computing and advanced analytics, is the key to extracting this value.
This article explores the critical steps in this transformative journey, moving "From Data to Decisions" by embracing the power of data integration, prioritizing data quality, and extracting maximum value through cloud-powered analytics and machine learning. This digital transformation is supported using cloud-powered analytical tools, which allow energy companies to optimize their assets, improve performance, reduce costs, and increase profits.
I. The Integration Imperative: Breaking Down Data Silos
One of the most significant hurdles facing energy and commodities firms is the fragmentation of data. Data resides in isolated silos across departments, legacy systems, and external providers. This fragmentation limits the ability to gain a comprehensive understanding of the market and make informed decisions.
The Problem: Silos prevent a holistic view of the value chain. For example, the impact of a weather event on the supply chain, demand, and pricing cannot be accurately assessed if weather data, production data, and market data are not integrated.
The Solution: Implementing a centralized, integrated data platform is crucial. This involves:
Data Integration: Implementing a centralized, integrated data platform, often built on a cloud infrastructure, is crucial.
Data Lakes & Data Warehouses: Establishing a central repository in the cloud to store all relevant data, structured or unstructured.
Cloud Data Pipelines: Developing automated data pipelines using cloud-native tools to extract, transform, and load (ETL) data from diverse sources.
APIs and Connectors: Utilizing APIs and connectors to facilitate seamless data exchange between systems, including third-party providers (e.g., weather services, market data vendors) and cloud services.
Cloud Computing's Strategic Role: Leveraging the inherent scalability, agility, and cost benefits of cloud-based platforms to manage the vast data volumes, complex processing requirements, and evolving analytics needs of the energy and commodities sectors. Cloud integration is crucial for integrating different sources.
Example: A large oil and gas company migrates its disparate data sources real-time sensor data from drilling operations, geological data, market price feeds, and weather forecasts to a unified cloud-based data platform. This platform facilitates real-time analysis, enabling faster decision-making and improved operational efficiency.
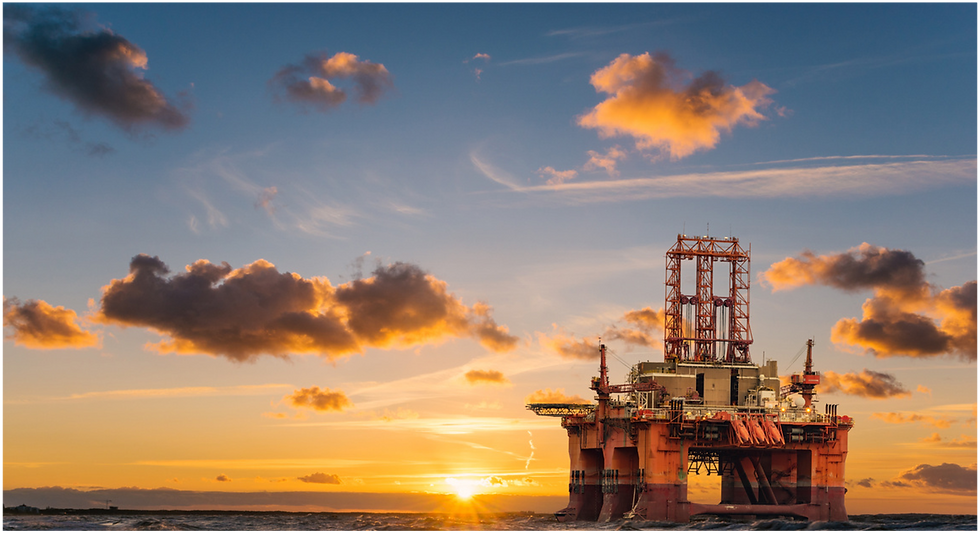
II. Data Quality: The Foundation for Trustworthy Insights in the Cloud
"Garbage in, garbage out" is a fundamental principle of data analytics. Poor data quality can lead to flawed models, inaccurate forecasts, and ultimately, poor business decisions. Energy and commodities firms must prioritize data quality initiatives to ensure that their insights are reliable and trustworthy.
The Problem: Data quality issues include:
Incompleteness: Missing data points
Inconsistency: Conflicting data across different systems
Inaccuracy: Errors in data entry or measurement
Duplication: Redundant data
Outdated data: Data that doesn't reflect the current reality
The Solution: Data Governance and Automation
Data Governance: Establishing clear data governance policies and procedures, with considerations for cloud security and compliance, to define data ownership, data quality standards, and data validation rules.
Data Profiling: Analysing data to identify data quality issues and patterns.
Automated Data Cleansing: Implementing automated processes for correcting errors, handling missing values, and standardizing data formats. Cloud-based solutions streamline and accelerate these tasks.
Automated Data Validation: Implementing automated data validation checks to prevent data quality issues from entering the system.
Master Data Management (MDM): Creating a single, trusted view of critical data entities, such as customers, products, and assets.
Example: A commodity trading firm uses cloud-based data quality tools to automatically validate the accuracy of market price feeds. These tools establish alerts to identify and correct any discrepancies or anomalies in the data, preventing potentially costly trading decisions based on inaccurate information.

III. Extracting Value: From Analytics to Actionable Insights
Once data is integrated and of high quality, the next step is to leverage advanced analytics techniques to extract valuable insights.
Key Analytics Applications:
Predictive Analytics: Forecasting energy demand, commodity prices, equipment failures, and market trends. Machine learning (ML) is crucial.
Prescriptive Analytics: Recommending optimal decisions, such as optimizing supply chains, hedging strategies, and trading positions.
Real-Time Analytics: Monitoring operations, detecting anomalies, and responding to changing market conditions in real-time, supported by cloud-based streaming analytics platforms.
Supply Chain Optimization: Optimizing logistics, inventory management, and transportation using cloud-based tools to reduce costs and improve efficiency. This source offers insights into supply chain optimization.
Risk Management: Identifying and mitigating risks, such as price volatility, geopolitical events, and operational disruptions, through cloud-based risk modelling and scenario analysis, leveraging AI and ML.
Digital Twins: Creating virtual replicas of physical assets for simulations and analysis that can run in the cloud.
Technology Enablers:
Artificial Intelligence (AI) and Machine Learning (ML): Building predictive models, automating decision-making, and identifying hidden patterns in the data.
Cloud-based Analytics Platforms: Providing the scalability, processing power, and agility needed to analyse large datasets, rapidly build and deploy models, and integrate insights into decision-making workflows.
Data Visualization and Business Intelligence (BI) Tools: Creating dashboards and reports in the cloud to communicate insights effectively to stakeholders.
Cloud-Native DevOps and MLOps: Enables agile development and deployment of analytical models, automating processes like data ingestion, model training, and real-time inference.
Extracting Value: Focus, Culture, and Iteration
Focus on Business Outcomes: Aligning analytics initiatives with specific business goals, such as reducing costs, increasing revenue, improving efficiency, and mitigating risks.
Data-Driven Culture: Fostering a data-driven culture where data is used to inform decisions at all levels of the organization.
Continuous Improvement and Agile Development: Employing agile methodologies to continuously monitor and evaluate the performance of analytics initiatives in the cloud and make rapid adjustments.
Example: A power generation company leverages a cloud-based predictive analytics platform to forecast energy demand. They optimize the dispatch of power plants, reduce operating costs, and use real-time data from various sources to make more informed decisions about energy production.

IV. The Human Element: Skills and Organizational Change
While technology is a critical enabler, it's crucial to remember that data analytics is not just about technology; it's also about people and processes.
Talent Acquisition and Development: Investing in data scientists, data engineers, and analytics professionals with the right skills and expertise.
Cross-Functional Collaboration: Breaking down departmental silos and fostering collaboration between data teams, business stakeholders and IT.
Change Management: Managing the organizational changes required to embrace a data-driven culture and implement digital transformation initiatives, emphasizing the need for strong leadership and a clear vision.
Education and Training: Providing training to employees to enable them to understand and use data and analytics effectively.
Examples:
Companies are creating digital customer experiences and leveraging cloud-based solutions to ensure more responsive and transparent services.
Digital tools are being used to improve operations and inventory management and are also creating new digital experiences that can engage customers in the design of their products (e.g., personalized product development).
The Future is Data-Driven
The energy and commodities sectors are undergoing a significant transformation, driven by the increasing availability of data and the power of advanced analytics. Companies that effectively integrate data from diverse sources, prioritize data quality, and extract maximum value from their data will be well-positioned to thrive in this new environment. By embracing these principles, organizations can move confidently "From Data to Decisions," unlocking new opportunities for growth, profitability, and resilience.
At Braingraph, we bring extensive experience in managing complex commodity market data and transactions, serving C-Suite stakeholders across energy and commodity trading markets for over six years. We specialise in integrating and analysing data from multiple sources, including major exchanges, over-the-counter (OTC) transactions, and broker platforms, ensuring seamless data flow and maintaining the highest standards of data quality.
Our solutions incorporate advanced data validation techniques, automated anomaly detection, and comprehensive audit trails to ensure data integrity across trading operations. Through our deep understanding of market structure and regulatory requirements, we help clients navigate complex reporting obligations while optimizing their operational efficiency.